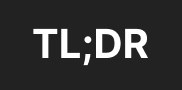
THE BIG IDEA
The arms race for training data is getting crazy
Do you have 8 trillion words of training data to spare?
That’s what it took to create DBRX, the world's largest open-source LLM, which portends a big issue on the horizon: According to the research institute Epoch, tech companies training new models could run out of high-quality data on the internet by 2026.
A bombshell report from The New York Times this week set the terms for the central conflict of the AI era: It’s a training data arms race on an absurd scale since more data makes better models. Call it Dune 2: The AI Edition, in which the increasingly rare spice is high-quality data that isn’t AI-generated.
The villains in this saga are Google, Meta, and OpenAI, which are pulling increasingly sketchy moves to bolster their models with high-quality data.
Let’s start with OpenAI. Remember when Mira Murati was so cagey about where OpenAI got all its training data a couple weeks back? Well, it turns out they scraped one million hours of YouTube videos, possibly violating the copyright of YouTube creators and Google’s terms of service.
But Google didn’t say anything. Why? Because they were also scraping YouTube videos and possibly also violating those creators’ copyright. Which sounds a lot like Omerta, the code of silence towards authorities held between warring mafia families.
The basic calculus seems to be that settling individual copyright lawsuits will be peanuts compared to the profits AI might generate. And negotiating licenses with publishers, artists, musicians, and the news industry would take too long and be far too expensive.
As if it couldn’t get more insane, Meta considered buying the publisher Simon & Schuster to use its books as training data in an effort to keep up. “The only thing that’s holding us back from being as good as ChatGPT is literally just data volume,” a Meta executive said. In the end, they seem to follow the lead of their Silicon Valley peers and scrape data from across the web.
Behind all of this, though, is a borderline existential threat to the current AI world order.
First, the Times report increases the chances that we’ll see successful copyright lawsuits against Big AI. If this comes with an order to pay creators for the use of copyrighted training data, the Big AI players admit it would ruin their business models and future plans.
Secondly, the fact that we’re running out of high-quality, human-generated text to use as training data — as Sam Altman has already readily admitted — is a real threat, even if training AI models on copyrighted data is deemed fair use. AI models need more data to advance. Right now, that training data needs to be human-created, since when AI models train on AI-generated text, you tend to see model collapse.
The only path forward is creating better synthetic (i.e. AI-generated data) that doesn’t cause model collapse, but there’s no guarantee we’ll get there. Meta, Google, and OpenAI will likely run out of training data by 2026 unless synthetic data improves. The suspense!
Even if AI isn’t good enough to write Succession, it’s giving us Succession-level plot lines.
CHART OF THE WEEK
Youngins are winning at AI

Nearly 1 in 3 Americans under 30 use ChatGPT at work, according to the latest Pew research. That’s up from 12% a year ago.
The generational difference is stark. There are also differences between education levels. Workers with graduate degrees are more likely to be comfortable using AI tools.
Just to put this all in perspective, 34% of Americans still haven’t heard of ChatGPT.
As Ethan Mollick pointed out, “The biggest AI opportunity companies are missing is that their employees are rapidly adopting AI & figuring out how to use it for work… and not telling leadership.”
WATERCOOLER
Amazon’s checkout AI was just a team of 1,000 people in India
Remember when it turned out that Theranos was just sending their blood tests to regular labs?
Well, Amazon’s amazing AI “Just Walk Out” checkout-free system at the Amazon Fresh stores turns out to have been staffed by a team of 1,000 people in India watching you shop on video, according to a report from The Information.
A spokesperson from Amazon disputed this, saying that the team in India mostly helps train the model. Yeah, OK, pal.
This is a wildly dystopian vision of our “AI-powered” moment: underneath the shiny surface is a creepy system of surveillance and outsourcing.
EXPERT CORNER
Bridging the gap between tech and healthcare
An interview with Richard Abrich, founder of OpenAdapt.AI, an AI-first process automation tool, and an expert in the A.Team Healthcare Guild.
Why did you first want to start finding AI solutions for healthcare?
I was studying computer engineering, and someone close to me had health complications that were exacerbated by an unnecessary, incorrect diagnosis. I was like, Why not use software to automate more of these tasks? Because if there had been some pretty basic processes in place, this might not have happened. And it's not just me. Basically everyone I talked to has had a bad experience in healthcare.
Healthcare is a uniquely challenging market for tech. Move fast and break things doesn’t work when lives are at stake. What’s your approach to reconciling that philosophical difference?
In my experience there are two things that you need to break into healthcare. One is you need to 10x the existing methodology. Like the fax machine which was ten times faster than snail mail. The other criteria is that you do something new that you couldn't do before. Like the MRI machines. Now, we can look inside people without opening them up.
With generative AI it seems very hard to make it work because you’re dealing with very specific data that you can’t afford to get wrong. What are the other challenges GenAI faces in healthcare?
One is hallucinations, as you said. The second is privacy. Right now, the state-of-the-art models are hosted by these private companies. There are open source models and they're catching up. But it seems inevitable that the closed models will outperform built in ones. But there's a third challenge: technical literacy. These technologies exist and are available, but hospitals don't have the resources to implement them. So it's not going to happen all at once.
What are the big opportunities you see?
There's a ton, right? A big one is diagnosis. This was what I spent my grad school on: Take an MRI or CT scan and predict if a person has cancer or diabetes. But there’s a disconnect between how technology works and how healthcare works. Geoff Hinton, one of the fathers of deep learning, famously said, We should stop training radiologists because in five years, we're not gonna need them. That was 10 years ago. Think of what a radiologist’s job is really about: They're not just diagnosing. They're also part of a system, and their job is to interact with other humans, other physicians, and other nurses in this system. So if you really want to replace them altogether, you need to basically build AGI. The best we can hope for today is a diagnostic aid.
How close are we to that?
We haven't really seen them proliferate because they don't meet that 10x criteria that I mentioned before. They’re expensive. They introduce all this other complexity. And maybe they’re increasing your throughput by 10 or 20%. So there's an opportunity but it has to be very targeted. It's not going to be surprising if these technologies are adopted in low-income regions because they simply don't have an alternative. That meets the second criteria. Before, they couldn’t get diagnosed at all.
EVENTS
Gen AI Salon: The Future of Health
If you found Abrich's commentary above interesting, you should come to our event at the end of the month focused on The Future of Health.
On April 24th we'll be hosting a Gen AI Salon in NYC with Carenostics Co-Founder, Kanishka Rao, Mount Sinai’s Rachel Tornheim, and Jessica Beegle, former Chief Innovation Officer at LifePoint Health.
We'll be diving into the big issues around implementation in one of the most risk-averse industries in the market. Come from the insights and behind the scenes intel. Stay for the witty banter.
DISCOVERY ZONE
Rewind is a personalized AI that tracks everything you do on your computer (slightly creepy but very useful!).
MEME
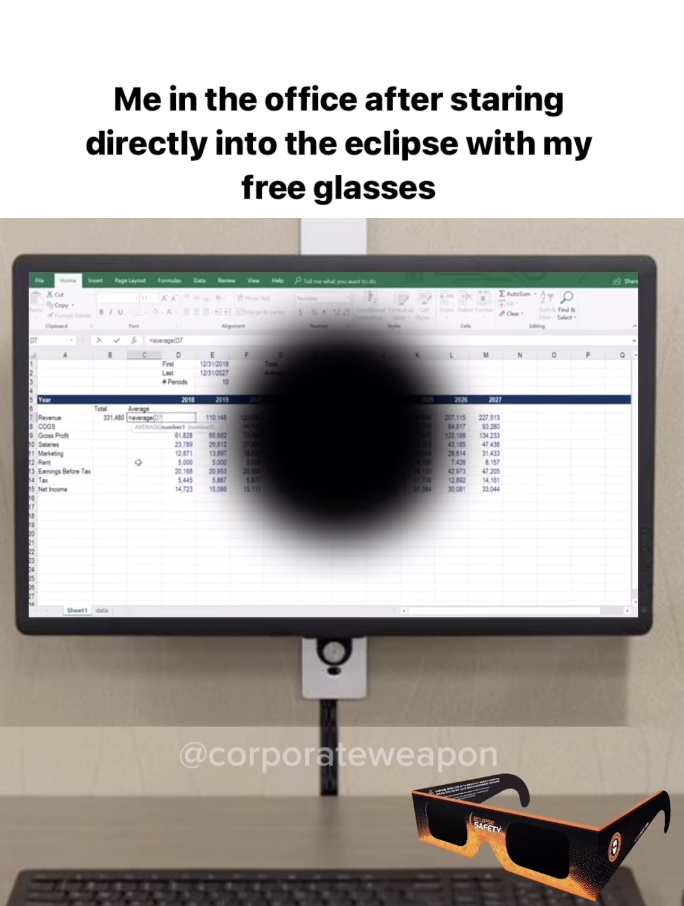